In recent days, the concept of "Agents" has become popular in AI space. This is not surprising, as automation has always been the goal of any technology. Every tech innovation tries to make things easier, and now with LLMs in the picture, any automated workflow with autonomous decision-making is basically what we call an Agent.
In this blog, we will explore what AI Agents are, and the essential coding agents every developer should know, and the frameworks used to build them.
Here is what’s in store:
What is NOT an Agent
What is an Agent
Understanding Tool Calling/Function Calling
Tool Calling in Real Time
Reasoning Models + Tool Calling
AI Agents Every Developer Must Know
Agent Frameworks
Tips for building effective agents
Let us begin with What is not an Agent,
What is NOT an Agent:
Let's clear something up, not every workflow with multiple LLM API calls qualifies as an Agent. Many folks get this wrong.
The diagram below shows a normal workflow where LLMs work with tools in a defined order. The LLM is not choosing what to do next, it is just following the path we have already defined.
What is an Agent:
An Agent is when we chain LLMs together and give them the power to decide what steps to take next, and how many steps needed to complete a task, instead of us instructing or defining prior what to do. The key difference is decision making autonomy. Below is the high-level diagram that shows the agentic workflow.
In short AI Agents is a system or workflow that use language models (LLMs) to make decisions and control the workflow and decide what happens next in a process to achieve the final result.
The tools you see here are functions or APIs, or anything that perform an action. They receive input from the LLM, perform their coded task, and return results back. The LLM then determines the next step based on these results. In AI terminology, when an LLM invokes a tool or function, this process is called function calling or tool calling.
Tool Calling / Function Calling
Tool or Function calling is the base of agents. The below high-level diagram shows tool calling with a single LLM. The LLM decides which tool to invoke based on the user's prompt and decides the next steps.
The LLM prepares the request in the format expected by the tool and then sends it. The tool works like an API. It processes the request and sends the result back. The LLM then decides the next step based on the results. This ability to autonomously select and call tools is what makes AI agents powerful.
When multiple LLMs collaborate, each managing different tools, it is known as a multi-agent setup.
Tool Calling in Real-Time:
Here are some screenshots captured during the stock analysis agent in action. You can see how it calls various tools, and the results generated.
I hope this gave you a better understanding of agents and tool calling. Agents can be designed for a specific purpose or built to handle multiple tasks, depending on our needs.
Reasoning Model + Tool Calling
All standard LLMs rely on tool calling as part of their routine normal workflow. However, with the emergence of Reasoning Models, that can think and plan step-by-step, the era of agents has truly begun.
So, why are autoregressive foundation models like GPT-2, GPT-3, and GPT-3.5 not commonly used in agents?
The reason is simple: Autoregressive models aren’t built for planning.
I came across an interesting point in a blog that if an LLM that is built on an autoregressive model, it lacks true reasoning and cannot plan steps. Meta’s Chief AI Scientist, Yann LeCun, has also stated that autoregressive LLMs can’t plan.
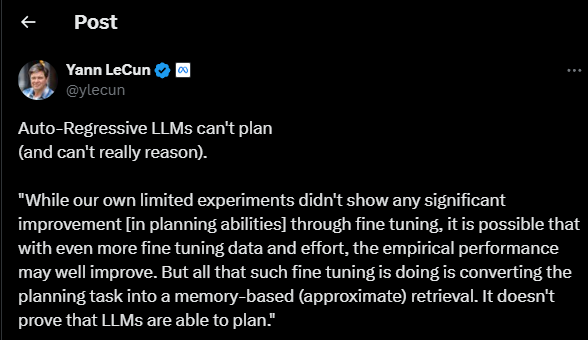
So now we get the point perhaps with the rise of Reasoning Models (like o1, o3, R1), agents have greatly enhanced their ability to plan and execute tasks more effectively.
After the basics of Agents, Now, let us explore some agents that every developer should know about.
AI Coding Agents for Developers:
We have coding agents that work in IDEs, browser-based, and some that run directly in the terminal.
Claude Code:
Claude code is an agent, which we can integrate with our terminal. It works with our code base and can perform below tasks.
Do coding and fix bugs in your codebase.
Execute tests and manage linting.
Understands code and do Q&A with your codebase.
Commit code to your Git, create PR, resolve merge conflicts.
For installation and more details on Claude Code, check their docs and GitHub Repo.
Note:- You need Anthropic’s API key to get Claude Code working for you.
I tried it on my codebase and have added sections and new page in the UI within minutes.
Claude Code is currently in Research Preview, so there are still bugs being reported in their GitHub repo, and some features may take time to fully develop. Try it out yourself to see how well it fits your workflow.
Some of the Terminal Commands, that you can use with Claude Code,
I also tried to commit the changes to GitHub repo, but it was not successful, but the way it instructs itself step by step to reach the destination is interesting. See below.
All the coding agents follow human-in-the-loop approach. It means they list the code changes and get the user approval to modify the code base.
Windsurf:
Windsurf has been making waves recently, so I decided to check out what kind of AI agent it brings to the table. Turns out, it has Cascade, an agentic bot which builds code and run commands based on user prompts and follows a human-in-the-loop approach.
Human in the loop approach is useful for automating workflows while still keeping some level of control. If you're curious about how it works, you can check out its agentic flow here.
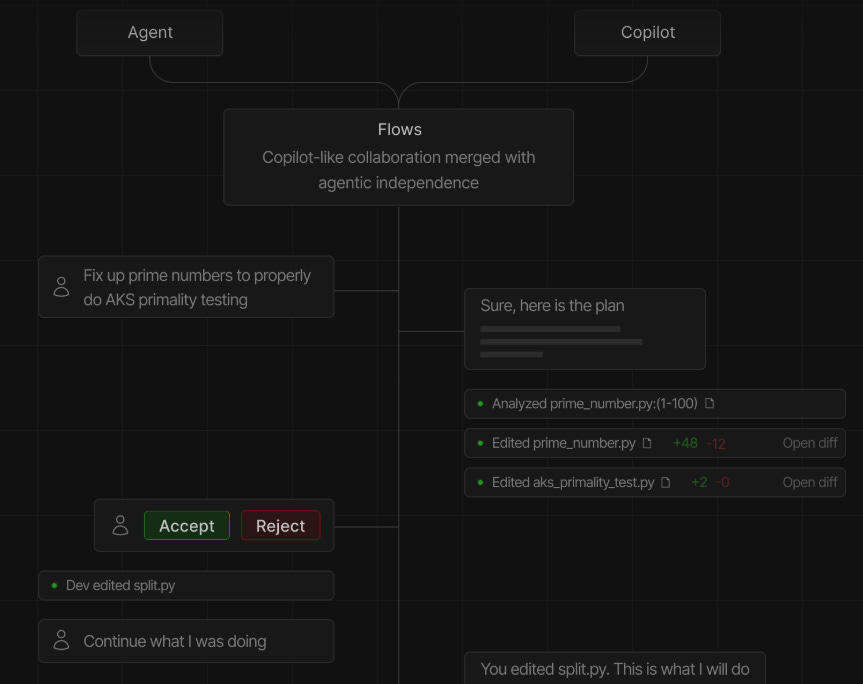
How to install and get started with Windsurf- Pls check here
With Windsurf, I completed an OAuth implementation in just 30 minutes for an existing project. Just dropped my credentials file in the directory and prompted the Agentic bot about cred.json
, and it started working. Remember when we'd spend 6-8 hours coding these OAuth flows manually? But here is how windsurf gets it done. And of course, I ran into a few issues along the way but fixed it by prompting Windsurf Cascade bot and completed the OAuth flow.
Windsurf and Cursor are pretty similar as they both are IDE based. Which one's better? Honestly, it depends on what you're trying to do. Everyone's workflow is different. You really need to try them yourself to figure out what clicks for you.
Mostly for frontend work, I go with any of these- Cursor, Windsurf, bolt.new, and lovable.dev. They're all crushing it lately. For the backend I always prefer Claude. Just experiment and see which one works better for you.
Cursor
Cursor, another famous IDE working as a coding agent. It has composer, a kind of chat interface, where agents work based on the user prompt, just like how Windsurf has cascade.
Cursor Agent can do below actions for you.
Reading & Writing code
Searching codebase
Call MCP servers
Run terminal commands
Automatic web search for up-to-date information
Want to try? Check the installation guide.
Note: Make use of cursor rules feature to set instructions for the Agent. These rules act like a system prompt allowing you to manage your code style, and architecture preference.
lovable.dev and bolt.new
lovable.dev and bolt.new are browser-based web development agent that let you generate and edit code using prompts without any local setup.
With Lovable.dev, you can push code directly to GitHub, deploy easily with Vercel or Netlify, and integrate with Supabase, though with some limitations. When using the Publish option, projects will be hosted on a Lovable.dev-generated URL by default, but a paid plan allows custom domain setup.
Bolt.new works similarly but has a key difference. It does not support direct GitHub push. Instead, you can download the code as a ZIP file and manually upload it to GitHub. However, it offers a built-in Netlify deployment, and at the end of a chat session, it provides a URL to transfer the project to your personal Netlify account.
The project that we are editing through Claude Code and Windsurf was initially built using lovable.dev. Below is a snapshot of the lovable.dev page, showing options to push code to GitHub, connect to Supabase, and publish as an app.
Below is the bolt.new screenshot, Check the end of chat, it gives the url to transfer the hosted project to personal Netlify account.
The next coding agent that we are going to see is Goose.
Goose:
Goose is an open-source AI agent available as a CLI tool and a desktop app (Mac-only). It can code, execute your project, debug, and even interact with external APIs autonomously.
Goose supports multiple LLM providers, giving you the flexibility to choose the best model for your workflow. It works well with Claude 3.5 Sonnet, OpenAI GPT-4o, and even offers a free tier with Gemini. You can check the full list of supported LLMs here.
Simplified Goose Architecture is below:
Tested goose with coding, web scraping and GitHub tasks, and it yes, it works.
Google Gemini offers a free tier for Goose allowing you to get started easily. Otherwise, you'll need to ensure that you have credits available for your LLM API.
After looking at coding agents, let's check out another useful tool, a code review agent. Since code review is as important as writing the code, let us see what Code Rabbit - A Code Review Agent offers in this space.
CodeRabbit: PR Review Agent
CodeRabbit is an AI Powered Code Reviewer, that works on pull requests and provide reviews in minutes. Are you a repo owner? Feeling hard to review your PRs every day? Give CodeRabbit a try.
CodeRabbit prioritizes data privacy and security. Here’s how it ensures that.
CodeRabbit does not use collected data for training.
Code is only stored in memory during reviews and is deleted immediately after. Only conversation and workflow embeddings (not code) are stored to improve future reviews.
Follows SOC2 Type II and GDPR standards to keep data secure and isolated.
All requests are encrypted via HTTPS.
It is easy to configure. Check the QuickStart Guide.
It can review PR and provide,
Summary,
Walkthrough and Sequence diagram,
Comments for every code change in the PR,
You can also chat with CodeRabbit Agent mentioning @coderabbitai in the comment section for any queries,
Attaching some of the reviews by CodeRabbit.
Hope you got some idea on how it works. Try integrating CodeRabbit and see how it works for you.
Now that we've explored Agents, let's look at the frameworks available to build them.
Agent Frameworks
Agent frameworks help developers build AI agents easily. They also come with ready-made agents for different tasks, like Code Agents for coding, Research Agents for gathering information, and Web Scraping Agents for extracting data from websites. You can pick the right agent based on what your project needs.
The word cloud below shows some of the most popular Agent Frameworks.
We will save a deeper dive into agent frameworks for a future post. For now, I'll leave you to explore them on your own and wrap up with some practical tips for building effective AI agents.
TIPS for building Effective Agents:
Design the workflow with strong error handling and retry mechanisms, allowing the LLM to self-correct when mistakes happen.
Keep it simple—a cleaner workflow reduces errors.
Avoid unnecessary LLM calls to improve efficiency.
Try reasoning models in workflow, instead of normal foundation models.
Even the best agentic workflows need constant evaluation to improve their chances of success. While evaluation is not strictly required for an agent to function, it is a key to making it truly effective.
Conclusion
I hope this blog has given you a clear understanding of agents, their framework, some popular agents, and how they work. Remember, knowing when to use or not use agents is just as important as knowing about them.
As AI-powered development grows, we will see more agents and frameworks shaping the way we work, making workflows smoother and more efficient. Try out different agents, see what they can do, and find the ones that fit your needs best.
Got any thoughts or experiences with coding agents? Drop them in the comments. Let us share knowledge!
Happy Coding!!!